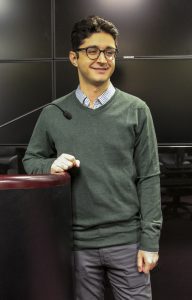
Dr. Ahmadreza Faghih Imani presented a UT-ITE seminar on February 8, 2019 entitled “Passive data for transportation modeling: imputing mode and purpose information from cellphone traces.”
According to Dr. Imani, lack of individual and trip information is a limitation of data collected passively, i.e. from cell phone use.
In particular, details on the mode of travel – whether car, transit, or active transportation such as walking or cycling – and the purpose of trips are not part of the available data.
Accurate transportation modelling requires this information.
Imani and his research colleagues solved this issue by creating high accuracy estimates of travel mode and trip purpose, using an innovative neural network approach.
For Dr. Imani’s full seminar, please see his presentation slides and/or a videorecording of his presentation.
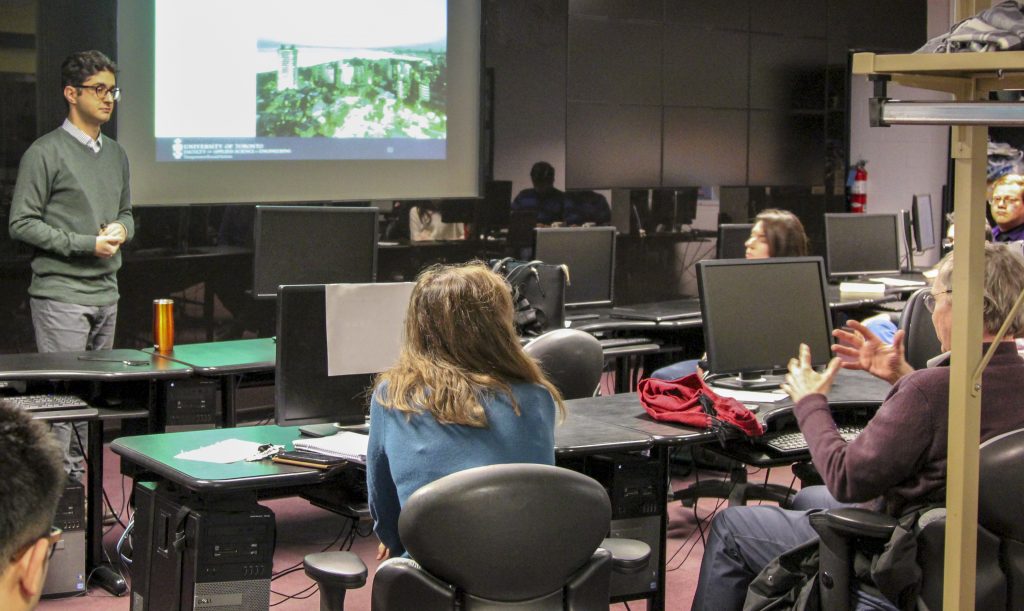
Abstract
In recent years, due to changes in communication, increasing costs, declining response rates and other methodological issues, new approaches are being sought to collect travel survey data.
One approach is to use cellphone traces collected from cellular network base transceiver stations (BTSs). The increasing market penetration of cellphones provides a very large sample of data with widespread coverage and low cost, especially for urban regions.
While cellphone trace data can be used to identify mobility patterns, the lack of trip information such as mode and purpose is a key limitation. Mode and purpose imputation is further complicated by technical limits to the spatial and temporal precision of the traces that can generally be achieved.
To date, the question of mode imputation has often been ignored, with researchers focusing on auto-based travel. In this study, household travel survey data and public transit smartcard transaction data are employed to help identify cellphone trace travel mode using a deep neural network approach. The empirical case study region for this research is Montevideo, Uruguay, where the high-quality data are available for all three types of data. Results obtained are very promising, with 98% correct predictions achieved by the trained model on its training set, and 87% correct predictions achieved with the validation set. Comparison of predicted cellphone trace travel modes with the observed household travel data yields reasonably good results. In addition, observing the travel patterns, home and work locations of cellphone users are identified. The final result thus is an enhanced representation of origin-destination trip-making by time of day, trip purpose and mode for the Montevideo region.
Speaker
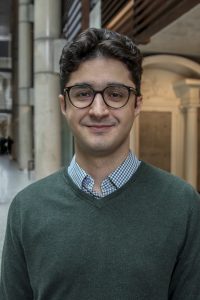
Ahmadreza Faghih Imani is an NSERC Post-Doctoral Fellow at the University of Toronto Transportation Research Institute. He obtained his PhD in Civil Engineering (Transportation) from McGill University in 2017. His dissertation on examining bicycle-sharing systems was recognized with the 2015 Benjamin H. Stevens Graduate Fellowship in Regional Science from North American Regional Science Association (NARSC). He is a young member of the Transportation Research Board’s (TRB) Committee on Bicycle Transportation.
This seminar was presented by the University of Toronto Institute of Transportation Engineers (ITE) Student Chapter.