Francisco Calderón Peralvo presented “Modelling Mobility Services within Agent-Based Travel Demand Model Systems and Implementation of a Ridehailing Case Study” on January 22, 2021 for the UT-ITE seminar series.
Conventional travel demand model systems simulate a typical day by looking at each vehicle, activity, type of transit, and travel distances. However, these models only consider demand and supply interaction. Given that ridehailing is a dynamic, complex service, Calderón adds a third dimension: service provision.
Modelling mobility services is challenging because these services are a moving target due to rapid growth, broad range of service types, and new services and technologies constantly emerging. These dynamic services use adaptive/responsive real-time operations with human-driven fleets and unprecedented levels of integration through Mobility-as-a-Service (MaaS) applications. To address these challenges, Calderón proposes a generic, flexible, overarching solution that could capture space-time dynamics, model operational activities, and model decision-making human drivers.
The generic service provision process considers user and driver activity to determine pricing and matching between drivers to customers. The service then rebalances the supply across the area in anticipation of high-demand patterns.
Modelling service provision involves interactions among MaaS, mobility services, service fleets, and driver activity with demand and networks used in conventional model systems. Matching algorithms used are greedy, centralized greedy, and Hungarian, in order of least optimal to most optimal. First-order models are used, looking at overall system metrics like number of trips, available vehicles, unmatched vehicles, and dormant vehicles. In addition, Calderón models performance vs wait time and unique drivers per hour.
The driver activity modelling framework generates driver activity logs under a sequential decision making method. Calderón models service provider activities like drivers leaving, effective fleet available, and predicting times for vehicles first entering the system. He also analyzes the three algorithms (greedy, c-greedy, Hungarian) over factors like problem size, trade offs among time intervals, and computation time. Hungarian is the optimal algorithm, computing the most accurate results but requiring the heaviest resources.
Calderón explored dynamic pricing mechanisms such as multiplicative surge pricing in which surge factors applied to total fares. He also discussed threshold-based mechanisms, in which thresholds set by the service provider trigger a surge factor. For both of these mechanisms, the main drivers are supply/demand ratios and fleet utilization coefficients.
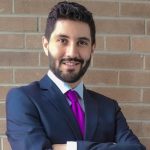
Dr. Francisco Calderón earned his Civil Engineering bachelor’s degree from the University of Cuenca, Ecuador and has design and field working experience in the areas of structures, geotechnical, and transportation. By 2017, Francisco obtained his Master of Engineering degree in Cities Engineering and Management, specializing in Transportation Systems, at the University of Toronto. Francisco continued his graduate studies pursuing a PhD in Transportation Modelling at the University of Toronto, under the supervision of Professor Eric Miller. His final thesis defense was held on November 2020. His main research focus consisted of modelling mobility services and Mobility as a Service (MaaS) within conventional agent-based microsimulation travel demand model systems, emphasizing on a supply-side perspective and detailed modelling of service provision processes.
Presented by University of Toronto ITE Student Chapter.
Related content
- Transformative Transportation ’20 – includes presentation “Modelling Mobility Service Provision” by Francisco Calderón and Professor Eric Miller, University of Toronto
- Travel Modelling Group presents workshop on mobility tools and services
- Mischa Young on the true cost of ridesharing