PhD candidate Seyed Mehdi Meshkani of the Laboratory of Innovations in Transportation (LiTrans) at Ryerson University presented “A dynamic many-to-one ride-matching algorithm for shared mobility services on congested networks” for the UT-ITE seminar series on November 5, 2021.
Meshkani began his presentation by introducing the increasingly popular transportation mode of on-demand shared mobility. Ride-sharing apps like Uber and Lyft use crowd-sourcing to offer passengers a ride directly from their smartphones. This is a promising and sustainable approach to mitigate externalities such as traffic congestion and vehicle emissions.
Meshkani explained that in spite of the benefits, there is still a need to develop well-designed systems that use the supply efficiently while providing a high-quality service.
Watch the presentation video recording
Abstract
On-demand shared mobility is a promising and sustainable transportation approach that can mitigate vehicle externalities, such as traffic congestion and emission.
On-demand shared mobility systems require matching of one (one-to-one) or multiple riders (many-to-one) to a vehicle based on real-time information. We propose a novel Graph-based Many-to-One ride-Matching (GMOMatch) algorithm for the dynamic many-to-one matching problem in the presence of traffic congestion. GMOMatch, which is an iterative two-step method, provides high service quality and is efficient in terms of computational complexity.
It starts with a one-to-one matching in Step 1 and is followed by solving a maximum weight matching problem in Step 2 to combine the travel requests. To evaluate the performance, it is compared with a ride-matching algorithm developed by Simonetto, et al. (2019). Both algorithms are implemented in a micro-traffic simulator to assess their performance and their impact on traffic congestion in the downtown Toronto road network.
In comparison to the Simonetto, GMOMatch improved the service rate, vehicle kilometre travelled and traffic travel time by 32%, 16.07%, and 4%, respectively. The sensitivity analysis indicated that utilizing vehicles with a capacity of 10 can achieve a 25% service rate improvement compared to a capacity of 4.
About the speaker
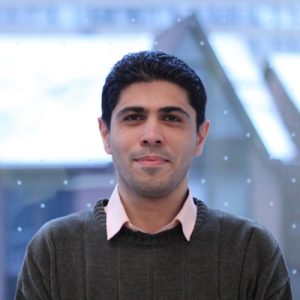
Seyed Mehdi Meshkani received his BSc degree (2010) from the University of Sistan & Baluchestan, Iran, and MSc degree (2013) in Roads and Transportation Engineering from Tarbiat Modares University, Iran. He is currently a PhD candidate at the Laboratory of Innovations in Transportation (LiTrans) at Ryerson University under the supervision of Dr. Bilal Farooq.
His research mostly focuses on designing on-demand shared mobility systems. More precisely, he develops matching algorithms for large-scale application and assesses their impact on transportation network utilizing simulation and optimization methods.
Presented by University of Toronto ITE Student Chapter, UT-ITE.